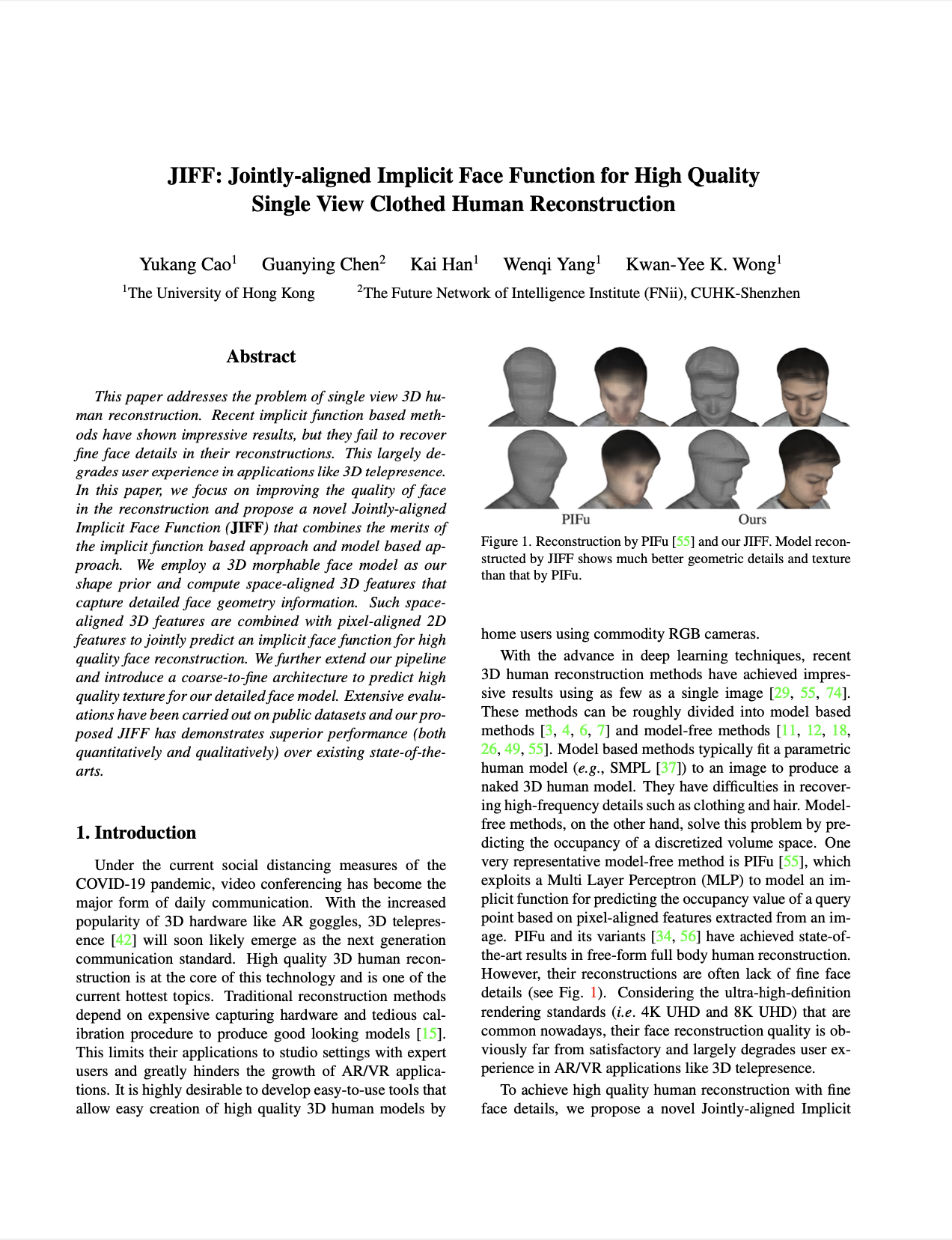 |
Y. Cao, G. Chen, K. Han, W. Yang, K.-Y. K. Wong.
JIFF: Jointly-aligned Implicit Face Function for
High Quality Single View Clothed Human Reconstruction.
In CVPR, 2022. [pdf]
@inproceedings{cao2022jiff,
author = {Yukang Cao and Guanying Chen and Kai Han and Wenqi Yang and Kwan-Yee K. Wong},
title = {JIFF: Jointly-aligned Implicit Face Function for High Quality Single View Clothed Human Reconstruction},
booktitle = {IEEE Conference on Computer Vision and Pattern Recognition (CVPR)},
year = {2022},
}
|